For many years already, artificial intelligence (or machine learning/deep learning) has been playing an important role in the area of IT security. It helps in the process of detecting anomalies in networks and tracking down intruders, it optimizes spam and virus filters, and it supports authentication procedures.
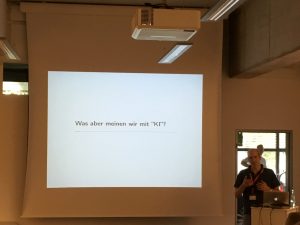
After a brief greeting by the Competence Group Leader Oliver Dehning, the CG meeting opened with a lightning talk by Claes Neuefeind from the Institute for Linguistics at the University of Cologne.
The presentation looked at artificial intelligence from a humanities perspective. In an initial foray into cultural history, it became clear that artificial intelligence has primarily been perceived as dystopian. Science fiction literature of the 1950s, along with Hollywood films, contribute to this, but early forms of artificial intelligence can be found in Goethe’s Magician’s Apprentice or in the mystical figure of Golem.
Regarding the terminology for AI, three important forms need to be differentiated:
• “Strong” artificial intelligence = emulation of a human
• “Weak” artificial intelligence = solving problems through the use of technology
• Machine learning = recognition, prediction, decision-making
Amongst the general population, AI is often understood as “mechanical thought”. “Thought”, however, means “conscious thought”, for which awareness is a prerequisite. But aware machines should be relegated to the realms of utopia.
When it comes to AI, nowadays it can generally be seen that attempts are no longer being made to create human-like machines, but rather to adapt humans to machines. Neuefeind cited bionics as an example of this.
Example use cases from industry
Following Neuefeind’s talk came example use cases on the topic of IT security and AI from industry. The first speaker was Ralf Benzmüller, whose specialist topic is “use of machine learning for the detection of phishing URLs” at G DATA. This methodology has been in use there for many years and improves the detection of Phishing attacks.
Up next was Peter Frey from eyeo, who uses machine learning for the detection of advertising on Facebook. Technically, this requires the interplay between image, text, and pattern recognition.
Thomas Hemker and Andre Engel from Symantec then took the stage. Here, machine learning has also been in use for many years for the detection of cyber attacks and is a component of many products, such as Intrusion Detection Systems and Threat Intelligence. Machine learning contributes significantly to improved detection and defense against cyber attacks.
The CG meeting was rounded off with a Round Table Discussion on the topic “What are the requirements of industry, in order to make Germany fit for the future in the area of AI?”
Big Data
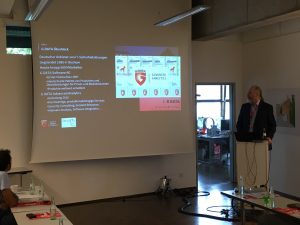
The basis for the machine learning described in all three use cases presented at the meeting is “Big Data”. Therefore, the availability of high-quality data sets represents a big challenge for industry.
One possible risk for the further development of AI research and innovation in Germany could therefore be data protection and the GDPR, which may develop into a competitive disadvantage.
The group was therefore excited by the idea of a GDPR-compliant, freely-accessible “Big-Data Pool” for the research and development of machine learning.
Remarks on IT security and cyber crime
In considering the area of IT security and cyber crime, participants at the round-table discussion observed the following:
• Cyber criminals continue to have data sovereignty, and there are now the first cases of attacks targeted specifically at sabotaging the training data of machine learning.
• The first attacks using artificial intelligence (DeepLocker) have already occurred and it is therefore also an urgent requirement that cyber defense be set up against such attacks.
• Speech-based AI, such as Alexa and Siri, reduce the security level of applications, and it is lagging behind biometric procedures. Examples of this are manipulations through ultrasound and voice manipulation. Several participants of the CG meeting see a strong security risk in the growing use of speech.
In some final comments, it was noted that, in general, there is a critical public perception of the term “artificial intelligence”, with this attributed to the fact that most use cases draw on simple machine learning. Furthermore, while the current research landscape in Germany and the use of machine learning in companies is cutting-edge, there is a lack of successful transferal from research into innovation. A last comment concerned the lack of specialist workers, with this regarded as representing an enormous risk for the future of artificial intelligence in Germany, not only in the context of IT security.